l
Development of Camera Calibration Field
The purpose of camera calibration is to
mathematically describe the internal geometry of the imaging system,
particularly after a light ray passes through the camera’s perspective center.
In order to determine such internal characteristics, a self-calibrating bundle
adjustment method with additional parameters is adopted [28,29] that can automatically
recognize and measure the image coordinates of retro-reflective coded targets.
Based on this functionality, we develop a rotatable round table surmounted by 112
pillars. The coded targets are then fixed to the top of the pillars and the
table surface to establish a three-dimensional calibration field with heights
varying from 0 to 30 cm. Instead of changing the camera location during image
acquisition, the table is simply rotated. Moreover, the camera’s viewing
direction is inclined 30°~45° with respect to the table’s surface normal. The
concept for the acquisition of convergent geometry by means of a rotatable
calibration field is illustrated in Figure 1. The round table is rotated at 45°
intervals while capturing the calibration images. This results in 8 images with
convergent angles of 60° to 90°, which is a strong convergent imaging geometry.
In order to decouple the correlation between IOPs and EOPs during least-squares
adjustment, it is suggested that an additional eight images be acquired with
the camera rotated for portrait orientation, i.e., change roll angle with 90°. Finally, for the purpose
of increasing image measurement redundancy, two additional images (landscape
and portrait) are taken with the camera’s optical axis perpendicular to the
table surface.
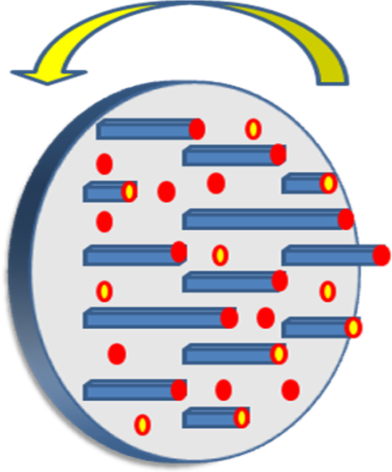
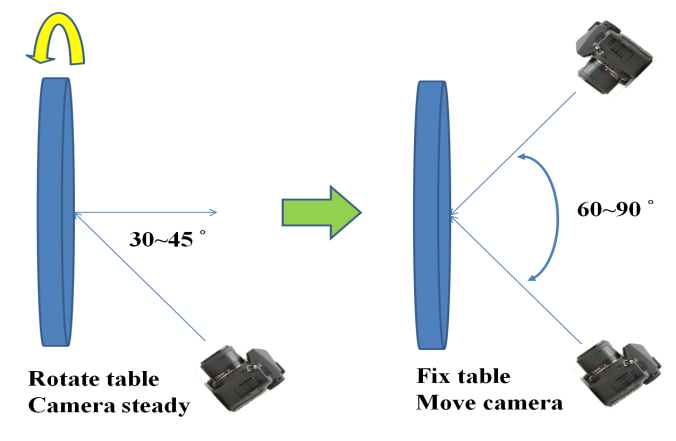
Figure 1.
Single camera calibration using a rotatable calibration field.
The relative position of all the code
targets is firmly fixed and remains stationary during rotation. This is
essentially the same as surrounding the calibration field and taking pictures,
introducing a ring type convergent imaging geometry. The proposed ring type
configuration is difficult to obtain with a fixed calibration field and
particularly for a limited space where the floor and ceiling will constrain the
camera’s location. A sample image for camera calibration is illustrated in Figure
2. One may observe that the coded targets are well spread out to the whole
image frame, especially the image corners, where the most critical regions to
describe the radial lens distortion are. In the figure, the bigger white dots
are designed for low resolution cameras to increase the number of tie-point
measurements by the auto-referencing function. The auto-referencing is
performed by predicting the detected white dots from one image to the others
using epipolar geometry in case the relative orientation has been established
in advance by means of code targets. Meanwhile, Figure 3 also shows two
enlarged code targets. In each coded target, two distance observables are
constructed by four white points and utilized for scaling purposes during
bundle adjustment. This means that the absolute positioning accuracy can
be estimated for the calibrated IOPs. Figure 3 depicts the result of bundle
convergence for one target from all cameras. The results demonstrate that it is
possible to obtain a strong imaging geometry by means of the proposed
arrangement.
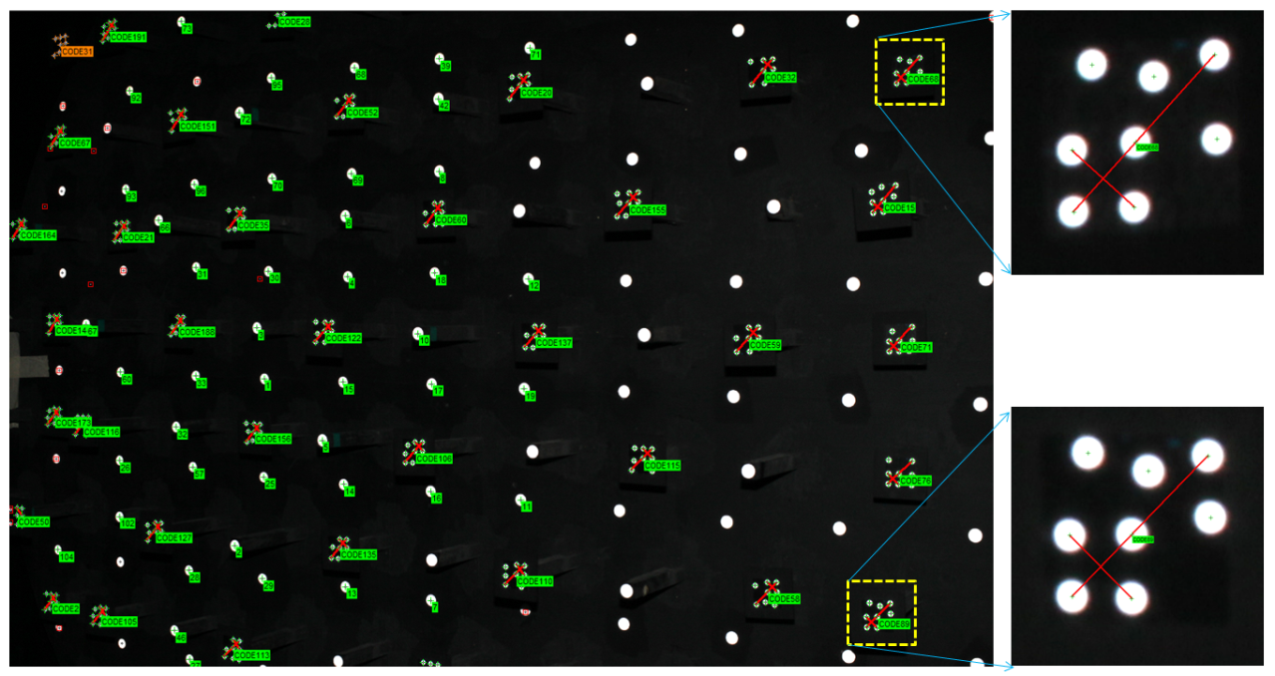
Figure 2.
A sample image for camera
calibration and two enlarged code targets.
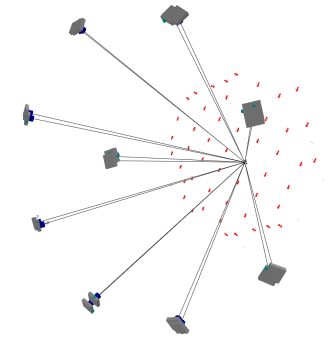
Figure 3.
Convergent bundles during
camera calibration.
l
Determination of Additional Parameters (APs)
Two approaches for determining the most significant
APs are suggested in this study. The first one is to check the change of square
root of the a posteriori variance (σ0)
value, which is a measure of the quality of fit between the observed image coordinates
and the predicted image coordinates using the estimated parameters (i.e., image residuals), by adding one
additional parameter at a time. The predicted image coordinates consider the
collinearity among object point, perspective center, and image point by
correcting the lens distortion. Thus, if a significant reduction in σ0
was obtained, for example 0.03 pixels, which is the expected accuracy of image
coordinate measurement by the automatic centroid determination method [30], the added parameter is considered as
significant one because it correct the image
coordinates displacement effectively. Otherwise, it can be ignored. This
procedure is simpler and easier to understand its geometric meaning when
compare with the next approach.
The second approach is based
on checking the correlation coefficients among the parameters and the ratio
between the estimated value and its standard deviation (σ), namely the
significance index (t). If two APs have a high correlation coefficient, e.g.,
more than 0.9, then the one with a smaller significance index can be ignored.
However, if the smaller one is larger than a pre-specified threshold, the added
parameter can still be considered significant. The threshold for the
significance index is determined experimentally, e.g., based on the results
from the first approach.
The significance index (t)
is formulated in equation (1), which is similar to the formula used for
stability analysis, as shown in Equation (2), namely change
significance (c), used for verifying the stability of a camera’s internal
geometry. Both formulations are based the Student’s test. The significance
index (t) is described as:
where δi is the
estimated value for parameter i and qi is the standard deviation for
parameter i [31], thus t has no unit. The variable t is an index
for the null-hypothesis that “the ith AP is not significant”
compared to the alternative hypothesis “the ith AP is significant”. On
the other hand, the change significance (c) can be described as:
where
; ∇i is the change of parameter i between calibration time
j and j + 1 and qi,j is the a-posterior variance of
parameter i at camera calibration time j [32], thus c has no unit as well. The variable c
is an index for the null-hypothesis that “the ith AP does not change
significantly” compared to the alternative hypothesis that “the ith
AP changed significantly”.
A SONY A-850
DSLR camera with SONY SAL50F14 (50 mm) lenses is adopted in
this study. The significance test results are summarized in Table 1 and can be
examined to illustrate the procedure for determining the most significant APs.
In the beginning, all APs are un-fixed during self-calibration bundle adjustment
to obtain the correlation coefficients between each other. Compatible with
common knowledge, the highest correlation coefficients occurred among K1, K2
and K3, i.e., greater than 0.9. In
the first run, we note that the significant indices for K2 and K3 are very low.
Since they are highly correlated with K1, they are both ignored. In the second
run, K2 and K3 are fixed at zero and the significant indices for P1 and P2 are
even lower than for the first run. Thus, they are ignored and fixed at zero at the
third run. At the third run, B1 and B2 still have significant indices of 29.7
and 15.8, respectively, which is difficult to determine their significant
level. Another approach is thus utilized to determine the significant APs by
adding one or two parameters and checking the change of σ0 after
bundle adjustment. In the lower part of Table 1, one may observe that a
significant improvement in the accuracy occurs only when K1 is considered. Even
adding K2, K3, P1, P2, B1 and B2 step by step, the σ0 has reduced
only 0.01 pixels, which is less than the precision of tie-point image
coordinate measurement, and the overall accuracy is only improved by 0.0014 mm.
This means that they can all be ignored by keeping only the principal distance
(c), the principal point coordinates (xp, yp), and the first radial lens
distortion coefficient (K1).
Table 1. Significance testing for
the determination of additional parameters.
run
|
items
|
c
|
xp
|
yp
|
K1
|
K2
|
K3
|
P1
|
P2
|
B1
|
B2
|
1
|
σ
|
0.0019
|
0.0025
|
0.0015
|
2.50E-07
|
1.30E-09
|
2.00E-12
|
2.30E-07
|
1.60E-07
|
6.40E-06
|
6.90E-06
|
Estimated Value
|
52.26282
|
0.120988
|
-0.05591
|
5.30E-05
|
7.37E-09
|
-1.90E-11
|
-3.79E-06
|
-2.64E-06
|
-1.98E-04
|
-1.03E-04
|
Significant
Index
|
27506.7
|
48.4
|
37.3
|
212.1
|
5.7
|
9.5
|
16.5
|
16.5
|
31.0
|
15.0
|
2
|
σ
|
0.0018
|
0.0026
|
0.0015
|
3.60E-08
|
4.30E-12
|
4.30E-15
|
2.40E-07
|
1.70E-07
|
6.60E-06
|
7.20E-06
|
Estimated
Value
|
52.25403
|
0.12224
|
-0.05537
|
5.32E-05
|
0.00E+00
|
0.00E+00
|
-3.69E-06
|
-2.54E-06
|
-2.01E-04
|
-1.02E-04
|
Significant Index
|
29030.0
|
47.0
|
36.9
|
1477.3
|
0.0
|
0.0
|
15.4
|
14.9
|
30.4
|
14.1
|
3
|
σ
|
0.0018
|
0.0013
|
0.0013
|
3.70E-08
|
4.40E-12
|
4.40E-15
|
4.40E-10
|
4.40E-10
|
6.80E-06
|
7.30E-06
|
Estimated Value
|
52.25684
|
0.087639
|
-0.06862
|
5.31E-05
|
0.00E+00
|
0.00E+00
|
0.00E+00
|
0.00E+00
|
-2.02E-04
|
-1.16E-04
|
Significant
Index
|
29031.6
|
67.4
|
52.8
|
1436.1
|
0.0
|
0.0
|
0.0
|
0.0
|
29.7
|
15.8
|
run
|
c
|
xp
|
yp
|
K1
|
K2
|
K3
|
P1
|
P2
|
B1
|
B2
|
σ0 (pixels)
|
Relative Accuracy
|
Overall Accuracy
|
1
|
■
|
■
|
■
|
|
|
|
|
|
|
|
2.00
|
1:6,200
|
0.3275
|
2
|
■
|
■
|
■
|
■
|
|
|
|
|
|
|
0.21
|
1:67,200
|
0.0303
|
3
|
■
|
■
|
■
|
|
■
|
|
|
|
|
|
0.21
|
1:67,800
|
0.0300
|
4
|
■
|
■
|
■
|
■
|
|
■
|
|
|
|
|
0.21
|
1:68,000
|
0.0299
|
5
|
■
|
■
|
■
|
■
|
|
|
■
|
■
|
|
|
0.21
|
1:68,600
|
0.0297
|
6
|
■
|
■
|
■
|
■
|
|
|
|
|
■
|
■
|
0.20
|
1:70,300
|
0.0289
|